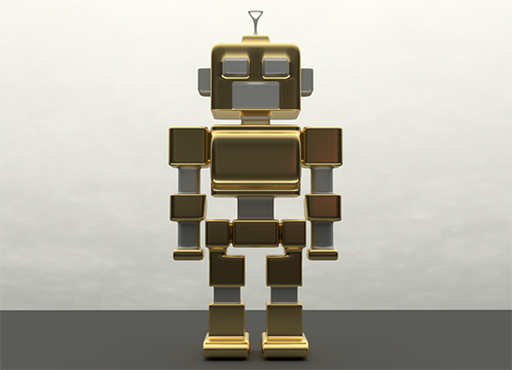
As a large language model, chatGPT has already shown a lot of potential for a wide range of applications. However, there are still many ways in which chatGPT and other language models are likely to evolve and improve in the future. Here are some potential directions for chatGPT development:
- Increased efficiency: One area of focus for chatGPT and other language models is likely to be increasing their efficiency. Language models require a lot of computational resources to run, which can be a barrier to their widespread adoption. As a result, there is a lot of interest in developing techniques and strategies to make language models more efficient, such as using more efficient hardware, optimizing the training process, or developing more compact models.
- Improved generalization: Another goal for chatGPT and other language models is to improve their ability to generalize to new tasks and contexts. While language models are able to perform well on a wide range of tasks, they still have difficulty adapting to new situations or handling tasks that require a deep understanding of context or background knowledge. Researchers are working on a number of approaches to address this issue, such as developing models with more flexible architectures, incorporating domain-specific knowledge, or using techniques like transfer learning to adapt models to new tasks.
- Enhanced interpretability: As language models become more widely used in a variety of applications, there is a growing need for ways to understand and interpret how they are making decisions. Researchers are working on a number of approaches to improve the interpretability of language models, such as developing techniques to extract more meaningful explanations from the models, or developing models with more transparent architectures that are easier to understand.
- Greater robustness: Another important area of focus for chatGPT and other language models is improving their robustness and resilience to adversarial inputs. Language models are vulnerable to a variety of attacks, such as adversarial examples or data poisoning, which can cause them to produce biased or misleading output. Researchers are working on a number of strategies to improve the robustness of language models, such as developing models that are more resistant to adversarial attacks or using techniques like data augmentation to make models more robust to changes in their input data.
- Integration with other technologies: Finally, chatGPT and other language models are likely to be increasingly integrated with a variety of other technologies, such as natural language processing (NLP), computer vision, and machine learning. This could enable a wide range of new applications and capabilities, such as language models that can understand and respond to visual input, or models that are able to learn from a combination of text and visual data.
Overall, there are many exciting directions for chatGPT development in the future, and it is likely that chatGPT and other language models will continue to play a central role in a wide range of applications. As these models continue to evolve and improve, they have the potential to transform a wide range of industries and applications, and to enable new levels of human-computer interaction and collaboration.